Leveraging Machine Learning to Predict Employee Attrition Risks and Guide Retention Interventions
How Machine Learning Can Help You Reduce Employee Turnover is a free webinar available on demand, presented by iQor’s Vice President of Operations Terri Robertson, Senior Vice President of IT Joe Przybylowski, and Data Scientist Andrew Reilly. Here’s an overview of what they discussed.
Why Retention Strategies Are Critical to Your Business Success
Employee attrition is a more pressing concern for organizations than ever, as evidenced by cultural dialogue like “The Great Resignation” and “quiet quitting.” Attrition is even more significant for BPOs like iQor whose business is to deliver outsourced customer service to the brands that entrust us.
The cost of employee attrition is significant in both direct and indirect ways. For example:
- The average cost to hire and train a replacement employee can be up to 200% of that employee’s salary.
- Replacing an experienced employee with a new hire can lead to a decrease in productivity and efficiency, which can affect revenue.
- A high turnover rate can lead to discontinuity in customer service, which can result in loss of business.
- High turnover can impact morale among other employees and cause a snowball effect of attrition, incurring further costs.
The value of investing in employees speaks for itself. At iQor, employee experience is a cornerstone of our business model. Creating a work environment that promotes job satisfaction, recognition, and growth opportunities is critical to retaining valuable tenured employees.
Using Predictive Analytics to Help Prevent Attrition With Proactive Retention Strategies at iQor
For over 15 years, iQor has used cutting-edge technology to provide an irresistible employee and customer experience (CX). Our team of expert data scientists has developed models for predictive analytics to guide our strategies for revenue recovery, customer satisfaction (CSAT), net promoter scores (NPS), and more. So, why not leverage our data analytics expertise to predict attrition before it has a chance to materialize?
Using the power of predictive analytics, we asked the fundamental question of attrition: How do you know when an employee is thinking about leaving?
To answer this question, our team of expert data scientists developed a machine learning model to identify variables that correlate with attrition and predict which employees might be at risk of leaving the company. Our goal was to play offense rather than defense to the problem of employee attrition by developing proactive retention strategies for at-risk employees. The model successfully:
- Analyzes which variables influence employee attrition.
- Identifies employees at risk of attrition.
- Guides our operations team’s retention strategies.
- Contributes to the retention of thousands of at-risk employees.
- Informs an improved employee experience through a culture of empathy and professional development opportunities.
Using the power of predictive analytics, we asked the fundamental question of attrition: How do you know when an employee is thinking about leaving?
Customized Employee Retention Processes Guided by Predictive Analytics
Predictive analytics placed the ball in our court to proactively shape a positive employee experience that improves retention. The foundation of machine learning and predictive analytics is data. To begin, iQor’s data scientists needed to know what data they required to build their “crystal ball” for proactive employee attrition prediction.
Their approach to data modeling consists of three stages:
1. What data is available?
The existing body of structured data could include tenure, compensation and benefits, workforce management data, interaction analytics, coaching outcomes, performance reviews, bonus history, training data, and other information that iQor already gathered about our employees.
2. What are we missing?
After conducting an inventory of existing data, we could identify data gaps. Gaps often include unstructured data, which is more difficult to gather and too subjective to easily evaluate, such as employee feedback.
3. What can we create?
Identification of data gaps can then drive strategies for gathering that data. For example, annual surveys for employee feedback are an option, but that doesn’t provide enough data for accurate analytics. Filling in this gap requires a high-frequency method of employee surveying for gathering reliable and timely feedback regarding their outlook on the job.
The answers to these questions create a broader data pool of structured and unstructured data to begin identifying which data points correlate to attrition risks.
Transforming Raw Data Into Usable Variables
Data is just raw material; it needs to be refined to be useful, as described by Gartner’s Four Phase Data Maturity Model. iQor’s data scientists had to decide how to categorize the data into variables that could be computed by their algorithms. They then validated each data feature using a process called variable importance testing to determine what to include and exclude from the production model. This process assesses how much influence a particular variable or feature has historically impacted our attrition. Those that rank high are selected while those that rank low are rejected.
Using these variables and a combination of five main algorithms, our data scientists deployed a five-fold cross-validated Stacked Ensemble Meta learner with auto-tuned hyperparameters—or, in layman’s terms, they created a customized employee retention model that could assess the probability of employee attrition within the next 60 days.
From Data Science to Pragmatic Action: The Plan for Proactive Frontline Employee Retention
At iQor, we place a high value on providing exceptional employee experiences. As our data scientists and operations teams collaborated to develop a practical, specific, repeatable process for proactive retention, they kept the employee experience top of mind. The process needed to be simple for employees and managers in terms of time and effort expended; it needed to be standardized yet flexible; and it needed to be embeddable into our feedback culture. The result was a Measurable Skip-Level Meeting, also known as “The Touch Base.”
“The Touch Base” Strategy for Employee Retention
Every week, iQor runs our machine learning model for all 40,000 employees company-wide. We analyze each individual’s probability of attrition between 0% and 100%. At-risk employees are identified at a threshold of 65%. Employees who meet or exceed this threshold for attrition risks will automatically engage the company’s proactive retention strategy enacted by the operations teams.
As a result of these analytics, our operations teams are informed of at-risk employees every week. They receive the employees’ names, the names of their direct supervisors, and the skip-level managers responsible for scheduling the Touch Base. These managers have a structured guide with clear, consistent guidelines, a system of record, and a method for feeding results back into the analytics model.
The Touch Base is standardized enough to provide data points that can guide improvements to the machine learning model but also conversational and customized to each employee’s needs.
It’s important to note that the Touch Base is not focused on the employee’s performance. Rather, it is about encouraging open communication to identify and support the employee’s needs. The anatomy of the Touch Base is as follows:
- Before the Touch Base
- The manager reviews the employee’s information to approach the conversation with an informed and empathetic mindset.
- The manager schedules the 30-minute session with the employee through our workforce management system.
- During the Touch Base
- The manager asks open-ended questions in an effort to identify what the employee may be experiencing that makes them at risk.
- The manager lets the employee guide the conversation to help the employee feel listened to, valued, and supported.
- The manager thanks the employee for their time and willingness to share.
- After the Touch Base
- We use the same module that guides our coaching to measure the effectiveness of each Touch Base. The Touch Base checklist includes a summary with the following priorities:
- Clarify timelines, deliverables, and expected outcomes.
- Agree on next steps.
- Schedule a follow-up meeting with the employee.
- Escalate to the next level of management, if needed.
- Lastly, the manager documents the meeting in the system with as many details as possible.
- We use the same module that guides our coaching to measure the effectiveness of each Touch Base. The Touch Base checklist includes a summary with the following priorities:
The manager also rates the Touch Base for their impression of the employee’s overall state of being. In some cases, just having the conversation with the manager can make the employee feel valued and clear up the risk of attrition. In other cases, the situation is not something the manager can easily address, and escalation may be necessary.
Combining Clinical Trial Methodology and Human Storytelling to Gauge the Effectiveness of the Touch Base Process and Guide Next Steps
For iQor’s machine learning model to be effective, it has to be able to measure the effectiveness of our interventions and the results of these Touch Base meetings. We performed a controlled study, much like a clinical trial, to go beyond the manager ratings to use a scientific approach to our evaluations.
Comparing Retention Outcomes Between Control and Experimental Groups Showed a 2.6x Increase in Retention for Touch Base Employees
Out of 100 at-risk agents, we withheld a small sample of at-risk agents to serve as a control group. They received no Touch Base interventions. The other 95% served as the experimental group and proceeded through our Touch Base intervention process. The results were clear. After 60 days, the control group experienced 18.3% attrition while the experimental group experienced 7% attrition.
This led to two main findings:
- The model appropriately identifies employees at risk of attrition.
- The intervention is meaningful.
At-risk employees who go through the Touch Base process are 2.6 times more likely to stay with the company than employees who do not receive proactive retention strategies. We refer to this as our Attrition Mitigation Factor. The takeaway? Our Touch Base strategy worked to effectively retain at-risk employees and embody our commitment to providing an outstanding employee experience.
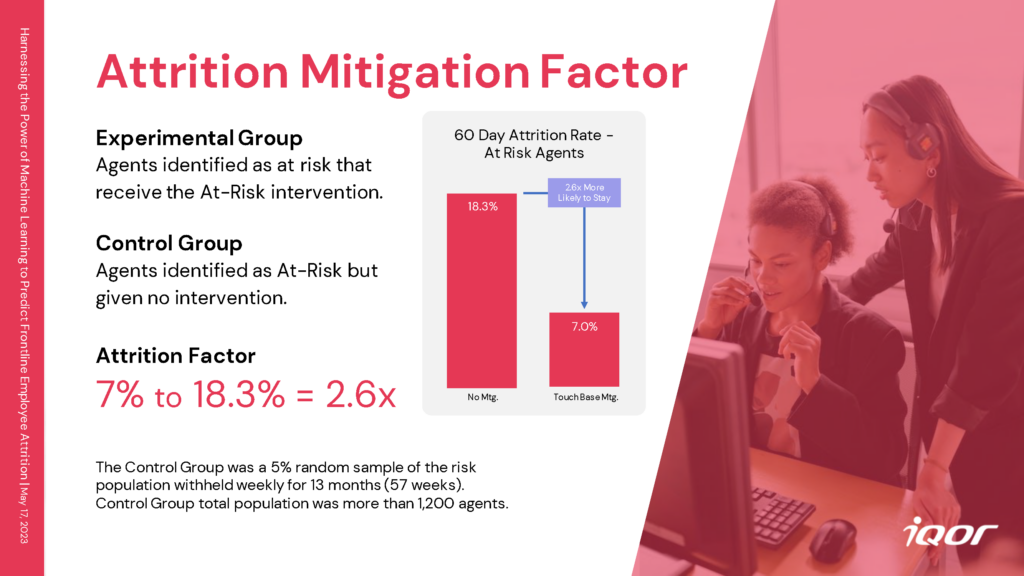
Stories Shared by Agents and Managers Through a Culture of Feedback Relate Positive Outcomes Beyond the Science
What steps actually work to retain at-risk employees? The answer to this can help us implement a repeatable process and create a culture of feedback. Feedback and storylines from our operations teams about their experiences with the Touch Base process guides continuous improvement to the model.
Using the Touch Base process, managers now get to talk directly to frontline employees instead of just hearing from their direct managers. This makes it much easier to find resolutions and improve relationships to encourage retention. When managers listen, they can facilitate adjustments that lead to employee retention. Sometimes, preventing employee attrition is as simple as adjusting schedules.
Putting the “Active” in “Proactive:” How the Machine Learning Model Constantly Self-Improves
iQor’s machine learning model is dynamic. Future improvements are guided by the data science team, the operations team, and stakeholders, who have the opportunity to provide feedback and ask questions during quarterly reviews. The key is data. Each time the machine learning model processes new information, it improves its own ability to fulfill its intended purpose.
Best Practices for a Machine Learning Approach to a Proactive Retention Strategy
iQor’s machine learning model is successful because our data science, operations, and frontline teams collaborated to define, implement, and improve the process. Embodying our iQorian Value of open communication helped our teams develop an effective machine learning model that kept the human experience at the forefront of the process.
Here are some best practices our teams followed:
- Define the problem in as much detail as possible through collaboration, data discovery, and analysis.
- Acquire high-quality data by asking three main questions: what data do we have, what data can we create, and what data can we enrich by joining it to another data source?
- Name the project to give it its own brand. Our Touch Base system makes the project memorable to stakeholders and builds value.
- Create a control group like a clinical trial to evaluate results for an accurate indication of effectiveness.
- List all KPIs to specifically define the model’s parameters.
- Start with a quick win to motivate involvement and maximize collaboration potential.
- Fail small and fast to allow the data science methodology to break the project into bite-sized pieces that are not overwhelming.
- Communicate between teams so they can seek support across the organization. Establishing a regular cadence for communication keeps channels open that we can use as potential input sources for continually improving the model.
Investing in Employees to Retain Tenured, High-Performing Teams
Employee attrition can be a significant issue for any company. Our proactive retention strategies help us stay ahead of the curve through processes that incentivize valuable employees to stay on board. iQor’s machine learning model successfully predicts attrition and guides effective intervention strategies, empowering us to transform at-risk employees into tenured career professionals that provide irresistible customer service.
Experience the Best in CX
iQor offers analytics as a service to enhance employee, customer, and client outcomes. Our proprietary speech analytics platform, cloud computing, machine learning, artificial intelligence, and data analysis enable us to provide effective workforce management solutions, flexible work environments, and improved coaching processes. We prioritize our employee experience and aim to cultivate a culture of success that fosters loyalty and high performance.
As a managed services provider of customer engagement and technology-enabled business process outsourcing (BPO) solutions, iQor provides a comprehensive suite of full-service and self-service scalable offerings that are purpose-built to deliver enterprise-quality CX.
Our award-winning CX services include:
- A global presence with 40+ contact centers across 10 countries.
- A CX private cloud that maximizes performance and scales rapidly across multiple geographies on short notice.
- A partnership approach where we deploy agents and C-level executives to help maximize your ROI.
- The perfect blend of intelligent automation for scale and performance coupled with an irresistible culture comprised of people who love to delight your customers.
- Virtual and hybrid customer support options to connect with customers seamlessly, when and where they want.
- The ability to launch a customer support program quickly, even when you need thousands of agents ready to support your customers.
- A best-in-class workforce management team and supporting technology to create a centralized organization that can better serve your entire business.
iQor helps brands deliver the world’s most sought-after customer experiences. Interested in learning more about the iQor difference? If you’re ready to start a conversation with a customer experience expert, contact us to learn about how we can help you create more smiles.
Joe Przybylowski is senior vice president of IT at iQor. Connect with him on LinkedIn.
Andrew Reilly is a data scientist at iQor. Connect with him on LinkedIn.
Terri Robertson is vice president of operations at iQor. Connect with her on LinkedIn.